Über uns
Die beste Finanzierung für dein Unternehmen – gefördert, flexibel, zukunftssicher!
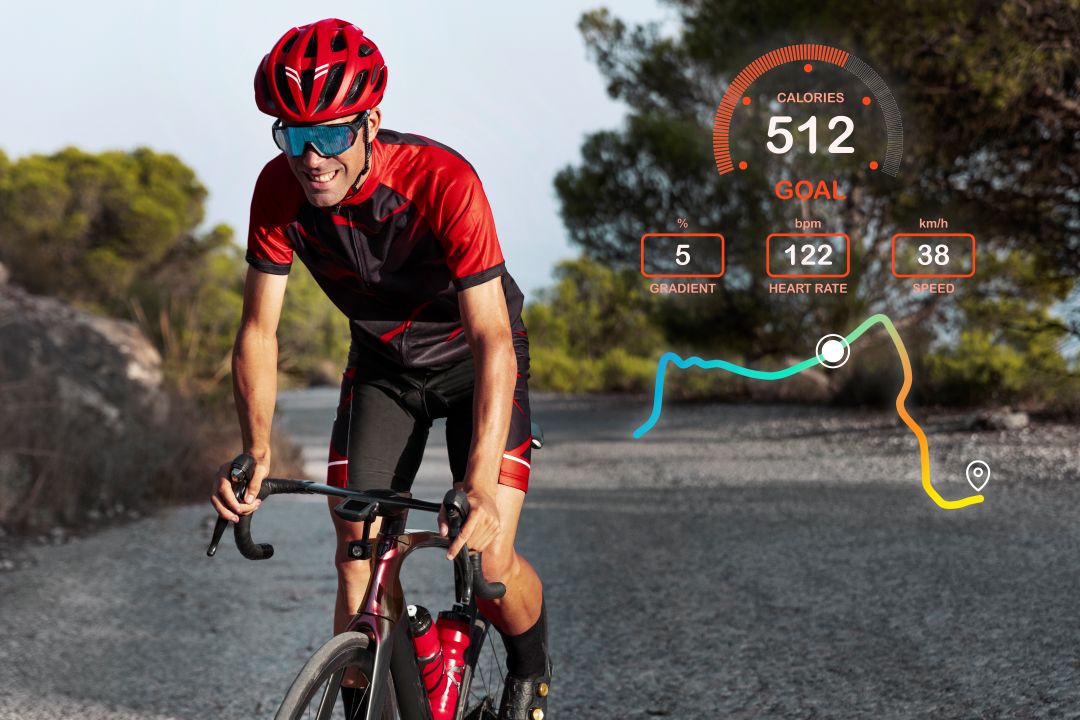
Wir sind die Agentur für smarte Finanzierungen und begleiten Unternehmen dabei, die optimale Finanzierungslösung zu finden. Durch gezielte Nutzung der österreichischen Förder- und Finanzierungslandschaft – mit Eigen- und Fremdkapital sowie Zuschüssen – ermöglichen wir unseren Kunden nachhaltiges Wachstum und Innovation.
STAND März 2025:
252
Projekte abgeschlossen
18+
Jahre Erfahrung
103+
Kunden
Kundenfeedback
Das sagen unsere Kunden
„Der Trackrecord in den letzten 6 Jahren ist enorm.„
Industriebetrieb
★★★★★
„Durch die Begleitung können wir verstärkt in die Entwicklung investieren“
Mittelständischer Halbleiterbetrieb
★★★★★
“Der Förderdschungel wurde durch die Zusammenarbeit endlich greifbar.”
Softwareunternehmen
★★★★★